Case Studies: Successful Personalization in Digital Marketing
Netflix
Netflix is a streaming service, the undisputed leader in the streaming industry and it owes much of its popularity to its personalized content suggestions or better, to its personalized recommendations.
By analyzing vast amounts of data, including viewing history, ratings, and even the time of day a user watches, Netflix employs sophisticated algorithms to deliver tailored recommendations to each individual user. At the heart of Netflix’s recommendation system lies machine learning and artificial intelligence.
Join the Course Today: https://erp.raznameh.org/register-on-marketing-course
Explore the Course Topics: https://erp.raznameh.org/slides/the-sales-and-marketing-playbook-15
By leveraging advanced deep learning techniques, such as graph neural networks, Netflix is able to extract meaningful patterns and insights from the vast sea of user data.
This technique analyzes user behavior and preferences to identify similarities between users and recommend content that similar users have enjoyed.
So, how does it work?
Netflix’s recommendation system relies on advanced artificial intelligence to deliver personalized suggestions to its users. This process begins by tracking various aspects of a user’s activity on the platform, such as the shows and movies they watch, how long they watch them, and whether they complete a series or film. Based on this data, Netflix builds a profile of each user’s preferences, identifying patterns in terms of preferred genres, themes, or even specific actors.
To refine recommendations, Netflix doesn’t just stop at individual viewing habits. It also considers how certain shows and movies are rated by users, both in terms of popularity and user satisfaction. The platform then compares this data with the viewing habits of other users who exhibit similar tastes, helping to surface content that may appeal to an individual user based on what others with comparable preferences have enjoyed. Additionally, Netflix’s algorithms assign scores to different pieces of content, taking into account factors like popularity, critical acclaim, and user feedback to evaluate how well each title matches a person’s unique viewing profile.
At the heart of Netflix’s personalization engine are sophisticated machine learning algorithms that analyze massive datasets to uncover trends and correlations that would be difficult for a human to identify manually. These algorithms are designed to adapt as new data is generated, continuously refining the recommendations by considering real-time user activity. In this way, the platform dynamically updates the list of suggested content, creating a custom experience tailored specifically for each viewer.
Therefore, by continuously refining its algorithms based on user feedback and interactions, Netflix ensures that its recommendations remain relevant and personalized.
Amazon
Amazon is a global technology company primarily known for its online retail marketplace, where customers can purchase a wide range of products, from electronics to books to clothing. Based on a customer’s shopping activity, Amazon reviews each customer’s preferences to create personalized recommendation types on their homepage and throughout the shopping journey, as well as provide personalized product descriptions that are more relevant to customers. The amazon product recommendation system is a cornerstone of the eCommerce giant’s success, continually evolving to provide unparalleled personalization. This sophisticated algorithm not only drives sales by suggesting relevant products but also enhances the overall shopping experience.
So, how does it work?
Its concept is fairly simple on the surface. Amazon’s AI-driven recommendation engine suggests products based on individual browsing history, past purchases, and items frequently bought together, significantly increasing the likelihood of sales. By analyzing product attributes and customer shopping information, like preferences, search, browsing, and purchase history, they leverage a Large Language Model (LLM) to edit a product title to highlight features that it believes are most important to the customer and their current shopping activity. To ensure these titles accurately reflect what matters most to each individual, another LLM, known as an evaluator LLM, challenges and improves the results, assuring customers see the best possible product information.
Amazon’s recommendation system, powered by its A10 algorithm, provides personalized product suggestions by processing large amounts of data. The algorithm’s primary goal is to understand user behavior and preferences to deliver tailored recommendations. This is achieved through two main stages: data gathering and data filtering, which enable the recommendation engine to develop a comprehensive view of both overall and individual customer patterns.
Data gathering involves two types of data: general information about products and users, and specific insights into relationships and dependencies between them. Understanding these connections allows Amazon to gain a deeper insight into customer purchasing behaviors. There are three main types of relationship help shape the recommendations:
- User-product relationship emerge when certain customer groups frequently purchase specific product types.
- Product-product relationship are identified when items share common features.
- User-user relationship arise when individuals with similar preferences display comparable buying habits.
In addition to these relationships, the algorithm uses various forms of data, including user behavior, demographic details, and product attributes, to further refine its recommendations. Then to create personalized shopping experiences, Amazon employs several data filtering techniques:
- Content-based filtering suggests products with similar characteristics to those the user has previously shown interest in.
- Collaborative filtering uses the collective experiences of users to suggest products.
By combining these methods, Amazon’s recommendation system continuously improves its ability to engage customers, drive sales, and create a personalized shopping experience.
Spotify
Spotify is a leading music streaming platform that allows users to listen to millions of songs, podcasts, and other audio content from a wide range of artists and creators. It uses sophisticated algorithms and machine learning to curate a highly individualized listening experience for each user.
So, how does it work?
One of the foundational elements of this personalization is the listening history. By tracking every song played, skipped, and saved, Spotify builds a detailed profile of a user’s musical preferences. If a user consistently enjoys certain tracks, this positive feedback is recorded; conversely, quickly skipping songs signals disinterest. Over time, this data paints a comprehensive picture of what each user loves to listen to.
Additionally, Spotify dives deep into the genre and artist preferences of its users. If someone frequently listens to pop music or has a favorite artist, the algorithm recognizes these patterns and starts to prioritize similar genres and sounds in future recommendations. This focused approach ensures that the music suggestions resonate with individual tastes.
Beyond just looking at what users like; Spotify’s algorithm analyzes the science of sound. This includes examining various musical attributes such as tempo, mood, and instrumentation.
As a result of this comprehensive data analysis, Spotify can create personalized playlists like “Discover Weekly” and “Release Radar.” These playlists combine familiar favorites with new, hidden gems, offering a fresh listening experience each week. By continuously learning from user behavior and adapting recommendations, Spotify ensures that it meets the evolving tastes of its listeners, keeping them engaged and satisfied with their music discovery experience.
Coca-Cola
Coca-Cola is a globally recognized beverage company, famous for its flagship product, Coca-Cola, a carbonated soft drink and one of the most iconic beverage brands in the world. In 2013, the company launched the “Share a Coke“ campaign, which revolutionized how brands engage with their audiences through personalization. The “Share a Coke“ campaign focused on replacing the traditional Coca-Cola logo on bottles with popular names, encouraging customers to find bottles with their names or the names of friends and family. This simple yet effective tactic transformed ordinary bottles into personalized experiences, inviting consumers to connect with the brand on a deeper level. By leveraging personal names, Coca-Cola fostered a sense of ownership and intimacy, prompting people to share their experiences on social media.
The success of the “Share a Coke“ campaign lies in its ability to tap into the emotional connections people have with names and personal relationships. By encouraging consumers to seek out bottles featuring their names or the names of loved ones, Coca-Cola effectively turned a mundane product into a fun and engaging experience. The campaign also featured names of various demographics, appealing to a wide audience across different age groups and cultures.
Additionally, Coca-Cola expanded the campaign by allowing consumers to customize bottles through online platforms and in-store kiosks, where they could create personalized labels with their names or custom messages. This further enhanced the personalization aspect, as consumers felt a sense of agency in designing their own products.
Coca-Cola’s “Share a Coke“ campaign exemplifies how personalization can be leveraged to create memorable marketing experiences that not only drive sales but also build emotional connections with consumer.
Glossier
Glossier is a beauty and skincare brand that evolved from the “Into The Gloss” blog, which engaged readers on beauty topics and product reviews. The brand focuses on minimalism and enhancing natural beauty. Glossier excels in personalization by directly involving customers in product development. They use customer feedback, social media comments, and reviews to refine existing products and launch new ones, creating a strong connection with their audience. The brand also personalizes online shopping by tailoring product recommendations based on user preferences and past purchases.
So, how does it work?
Glossier’s personalization efforts are rooted in a customer-centric approach. They continuously engage with their audience on social media to collect feedback, using insights to guide product development and updates. For instance, they often ask their followers about their skincare needs, product preferences, or packaging suggestions. This real time input influences not only product formulation but also marketing strategies. On the e-commerce side, Glossier offers a personalized shopping experience by recommending products based on customer’s previous purchases, browsing history, and skin type, which customers can specify on the website.
Moreover, Glossier utilizes user-generated content for marketing, showcasing real customer’s reviews, photos, and experiences on social media and their website. This not only personalizes the brand’s image but also makes their marketing more authentic. Personalized email campaigns are another key aspect, where Glossier sends targeted messages with relevant product suggestions and exclusive offers based on individual user activity.
Magic Spoon
Magic Spoon is a cereal brand that offers a modern take on classic breakfast cereals, designed for adults who prioritize health. Their products are high-protein, low-carb, and gluten-free, appealing to consumers seeking healthier options. Magic Spoon leverages personalization through its direct to consumer model, collecting data on customer preferences to recommend flavors and bundle options. Their playful branding also extends to personalized email marketing campaigns, which use customer purchasing history to deliver relevant product suggestions and promotional offers.
So, how does it work?
Magic Spoon’s personalization focuses on their direct to consumer model, where they collect data to tailor customer experiences. When new customers visit the Magic Spoon website, they can take a quiz that collects information about their dietary preferences, favorite flavors, and health goals. This data allows Magic Spoon to offer personalized cereal recommendations and bundle deals. Customers also receive follow-up emails based on their quiz responses , purchase history, and browsing behavior.
Magic Spoon continues to personalize through targeted retargeting ads, showing customers flavors they’ve explored or products that complement their previous purchases. They also segment their email campaigns based on customers’ dietary preferences, providing personalized content, such as recipes that include their cereal, along with relevant health tips. This approach helps make their communications more engaging and relevant to each customer’s lifestyle.
Ethical Considerations in Personalization
The ability to access vast volumes of data has far-reaching repercussions for society. With technological advancements, the human species today has the potential for solving more ethical issues of data collection and attaining even more accomplishments than ever before.
However, it is critical to remember that privacy, trust, and security are interconnected, as are law and ethics. In the realm of big data and advanced analytics, the topic of data ethics has emerged as a cornerstone issue. Data ethics refers to the moral obligations and best practices associated with collecting, handling, and using data, especially personal and sensitive information. As we navigate through an increasingly data-driven world, the ethical implications of ethics and data collection and usage are gaining prominence. This focus is not only a matter of legal compliance but also of building trust and maintaining a responsible image in the eyes of consumers and the public at large.
The importance of ethical data collection in today’s environment cannot be overstated. With businesses and organizations relying heavily on data to drive decisions, innovate, and offer personalized services, the way this data is gathered and utilized holds significant consequences. Ethical data collection ensures respect for individual privacy, prevents misuse of sensitive information, and upholds the principles of fairness and transparency. In an age where data breaches and misuse can lead to severe repercussions, ethical practices in data handling have become a crucial aspect of corporate responsibility and customer trust.
Data ethics, in the context of data collection and analysis, encompasses a set of values and moral principles guiding how data is collected, shared, and used. It involves considering the rights and privacy of individuals whose data is being collected and ensuring transparency and fairness in data handling processes. This field intersects with legal compliance, such as adhering to data protection regulations like GDPR, but it extends beyond mere legal obligations, delving into the realm of moral responsibility. Ethical consideration involves reflecting on the moral aspects and implications of decisions, actions, and practices, ensuring they align with accepted moral standards and principles such as fairness, respect, responsibility, and integrity. Therefore, when implementing personalization strategies, businesses must navigate several ethical considerations to maintain trust and ensure responsible use of customer data.
Transparency is paramount; companies should clearly communicate how they collect, store, and utilize customer data. Providing consumers with a straightforward explanation of data practices helps build trust and allows customers to make informed decisions about their privacy. For instance, many organizations include privacy policies on their websites and regularly update them to reflect changes in data usage.
Consent management is another critical factor. Organizations must obtain explicit permission from customers before collecting or processing their personal information. This practice not only aligns with legal regulations like GDPR (General Data Protection Regulation) and CCPA (California Consumer Privacy Act) but also empowers consumers by giving them control over their data. Effective consent management systems allow customers to opt in or out easily, ensuring that their preferences are respected.
Avoiding bias in algorithms is essential to ensure that personalization models are inclusive and equitable. Data-driven algorithms can inadvertently reinforce existing biases if the data they rely on is skewed. Companies should strive to create diverse datasets and regularly audit their algorithms to identify and mitigate any biases that may arise. This approach fosters a more inclusive personalization strategy that resonates with a broader audience.
Lastly, data security is a crucial ethical consideration. Protecting customer data from breaches and unauthorized access is vital for maintaining consumer trust. Organizations must implement robust security measures, including encryption, secure storage solutions, and regular security audits. By prioritizing data security, companies can mitigate risks and reassure customers that their information is safe.
Trends in Personalization
As personalization continues to evolve, several trends are shaping the way businesses interact with their customers. These trends not only highlight the importance of understanding individual preferences but also demonstrate the growing role of technology in delivering tailored experiences. Here’s a detailed exploration of some of the most significant trends in personalization today.
1. Hyper-Personalization
The advancement of artificial intelligence (AI) technologies continues to redefine marketing boundaries. Hyper-Personalization is one of the leading trends, which takes personalization to the next level by leveraging artificial intelligence (AI) and machine learning.
For salespeople at digital marketing agencies focused on highly personalized sales outreach, grasping these tools is critical for propelling their strategies into the next era of digital marketing. Hyper-personalization elevates personalization to an unprecedented level. It uses real-time, behavior-driven data from users to create interactions that are not just relevant, but context-specific. Paired with generative AI, it allows a brand to shift from merely reacting to consumer behaviors, to predicting and adapting to them.
Personalized sales outreach not only resonates more with potential clients but also significantly boosts conversion rates. Customers now expect tailored experiences that recognize their unique needs and preferences, and this expectation can make or break a sale.
To meet these demands, sales teams are increasingly leveraging generative AI, a technology known for its ability to learn from data patterns and make autonomous decisions. Generative AI enables the creation of ultra-personalized content that dynamically responds to customer behavior. This approach goes beyond traditional personalization, allowing businesses to offer a truly one-to-one experience that connects with each individual customer on a deeper level.
The rise of hyper-personalization, powered by generative AI, is revolutionizing the sales outreach landscape. This combination of advanced technology and tailored engagement strategies is transforming how brands interact with both prospects and existing clients. By utilizing these tools, businesses can reshape their digital outreach strategies, leading to improved return on investment (ROI) and fostering stronger customer relationships.
Moreover, leveraging generative AI and hyper-personalization can significantly enhance the customer experience. It allows for seamless and intuitive interactions that drive loyalty and retention, ultimately contributing to revenue growth. As the sales landscape evolves, businesses must stay ahead of the curve by embracing these innovative technologies and strategies.
2. Omnichannel Personalization
Omnichannel personalization is a transformative approach that tailors each customer’s experience to their unique needs and preferences across various touchpoints. This strategy extends beyond traditional personalized marketing, ensuring that customers receive relevant, contextual, and timely interactions across multiple channels, such as websites, mobile apps, and email communications.
To effectively implement omnichannel personalization, businesses must first unify their customer data. This involves aggregating information from various sources—digital and in-store interactions alike—into a comprehensive view of the customer. By utilizing a Customer Data Platform (CDP), companies can overcome data silos, allowing them to consolidate crucial customer details such as contact information, demographics, purchase histories, and engagement metrics. This 360-degree view empowers marketers to understand their customers better, enabling data-driven decision-making that enhances personalization efforts.
Once the data is unified, the next step is to segment audiences effectively. Gone are the days when marketers relied solely on basic demographic characteristics for segmentation. Today’s advanced artificial intelligence and segmentation tools allow businesses to create more nuanced audience segments based on predicted behaviors. With access to over 120 different traits, businesses can refine their targeting efforts, ensuring their outreach resonates deeply with each customer.
Achieving a unified customer experience requires activating customer data across multiple channels. This means delivering the right message to the right person through the appropriate channel at the optimal time. By leveraging an enterprise marketing solution that offers broad channel support and built-in personalization capabilities, businesses can streamline their marketing efforts. Using a single platform simplifies workflows, reducing the inefficiencies associated with managing multiple disconnected systems.
Ultimately, omnichannel personalization allows businesses to create seamless and meaningful experiences for their customers. By effectively unifying data, segmenting audiences, and activating insights across multiple channels, companies can enhance customer satisfaction, drive conversion rates, and foster long-term loyalty. As the marketing landscape continues to evolve, embracing this comprehensive approach is essential for staying competitive and meeting the demands of today’s consumers.
3. Voice-based Personalization
Voice-based personalization is rapidly becoming a game-changer in the realm of digital marketing, enabling brands to create more intuitive and customized experiences through voice assistants like Amazon’s Alexa, Apple’s Siri, and Google Assistant. This approach leverages the capabilities of these smart devices to deliver tailored content and services, fundamentally transforming how consumers interact with brands.
Their rise in popularity can be attributed to their convenience, efficiency, and the personalized experiences they offer. As voice assistants become more integrated into daily routines, understanding their functionalities and the impact on personalization strategies becomes essential.
At the heart of these voice assistants lies advanced speech recognition technology, which accurately interprets spoken commands. This involves converting audio input into text and processing it to execute the desired action. Over the years, improvements in this technology have enabled these devices to comprehend complex commands and recognize various accents and languages, making them more user-friendly.
Natural Language Processing (NLP) is another crucial component that enhances the interaction between users and voice assistants. Once a command is converted into text, NLP algorithms interpret the meaning behind the words, allowing for more conversational exchanges. This capability enables voice assistants to understand context, identify keywords, and extract relevant information, making interactions feel more intuitive and human-like.
The true power of voice assistants lies in their ability to provide personalized experiences. They can learn and adapt to individual users, remembering preferences, past interactions, and making tailored recommendations. This adaptability fosters a deeper connection between users and their devices, ultimately enhancing customer satisfaction and loyalty.
Integration with third-party applications and devices further expands the capabilities of voice assistants. Users can control smart home systems, order food, or book rides using simple voice commands, streamlining their daily tasks. This integration not only simplifies user interactions but also creates opportunities for businesses to engage customers in innovative ways.
The growing popularity of voice assistants is largely due to their ability to simplify tasks and enhance convenience. Users can perform various activities hands-free, from setting reminders to controlling smart home devices, which saves time and allows for multitasking. Furthermore, the natural language processing capabilities make these assistants accessible to a wider audience, including those who may not be tech-savvy.
4. Predictive Personalization
Predictive personalization is a sophisticated approach that leverages artificial intelligence (AI) to forecast future customer behavior based on historical data. By analyzing historical data, businesses can anticipate market dynamics and tailor their offerings to align with consumer preferences. This integration of advanced technologies has become essential for companies seeking to navigate the complexities of today’s fast-paced market environment.
At the heart of predictive personalization lies the use of AI-driven predictive analytics, which allows organizations to make informed strategic decisions. By analyzing vast datasets, AI algorithms can detect patterns in consumer behavior, enabling businesses to predict future trends with remarkable accuracy. As consumer behavior increasingly shifts due to factors like economic conditions and social media influence, understanding these dynamics is crucial for businesses aiming to stay competitive.
Predictive analytics relies on the collection of diverse data sources, including sales records, customer interactions, and social media metrics. High-quality data is essential for developing robust predictive models. Businesses must ensure that the data they collect is accurate, relevant, and up to date to generate meaningful insights. These algorithms analyze historical data to identify trends and correlations. Machine learning continuously improves its performance by learning from new data, thus refining predictions over time.
NLP plays a critical role in understanding consumer sentiments by analyzing unstructured data such as customer reviews and social media posts. By extracting insights from this data, businesses can enhance their predictive models, enabling more accurate forecasting of market trends. This subset of machine learning uses advanced neural networks to model complex patterns in large datasets. Deep learning algorithms can process vast amounts of data and identify intricate trends that traditional methods might overlook, thereby enhancing the accuracy of predictive analytics. The integration of predictive analytics and artificial intelligence (AI) offers significant advantages for businesses aiming to enhance their operations and customer interactions. One of the primary benefits is the enhanced customer experience.
By accurately predicting customer needs and preferences, companies can create personalized experiences that resonate with their audience. Tailored recommendations not only foster increased engagement but also contribute to higher levels of customer satisfaction, ultimately leading to improved loyalty.
Another critical advantage is the increase in sales and conversion rates. Predictive personalization allows businesses to refine their marketing strategies by delivering relevant product suggestions at opportune moments. When customers encounter tailored recommendations that align with their interests, they are more likely to make purchases. This strategic approach results in higher conversion rates, demonstrating the effectiveness of using data-driven insights to inform marketing efforts.
Additionally, predictive analytics enhances operational efficiency. By accurately forecasting demand, businesses can optimize their inventory and supply chain management processes. This capability leads to reduced operational costs and better resource allocation, contributing to an overall improvement in customer satisfaction. The ability to anticipate market needs allows organizations to maintain optimal stock levels and respond more swiftly to fluctuations in demand.
5. Zero-Party Data
Incorporating zero-party data into personalization strategies is becoming increasingly vital for businesses seeking to enhance customer relationships. Unlike traditional data collection methods, zero-party data is information that customers willingly share, offering insights directly from their preferences and intentions. This method has gained prominence as businesses pivot towards transparency and trust in data usage, especially in light of evolving privacy regulations and consumer expectations.
One of the key advantages of utilizing zero-party data is its accuracy. Since this data is provided directly by customers, it tends to be more reliable and up to date than information gathered from third-party sources. This high fidelity allows businesses to craft more tailored and relevant customer experiences, leading to better personalization of services and offerings.
By engaging customers in a way that feels natural—through quizzes, surveys, and interactive content—companies can foster an environment where users are encouraged to share their insights, thus building a more comprehensive understanding of their audience.
Furthermore, zero-party data aligns seamlessly with current trends in personalization, especially as companies move away from tracking customer behaviors without consent. With stricter regulations on data privacy, such as the phasing out of third-party cookies by major tech companies, leveraging zero-party data presents a viable alternative. It not only ensures compliance with these regulations but also enhances the customer experience by making them feel valued and understood.
The integration of zero-party data collection methods into marketing strategies—such as interactive tools, preference centers, and engaging content—can significantly improve customer engagement. When customers feel that their opinions matter and that they are receiving personalized attention, their loyalty and satisfaction tend to increase.
Thus, the adoption of zero-party data not only supports compliance with privacy laws but also fosters a more meaningful connection between brands and their customers, ultimately driving long-term loyalty and business growth.
Future of Personalization in Digital Marketing
As we navigate an increasingly digital landscape, the future of personalization in marketing is more dynamic and complex than ever. The ongoing evolution of technology and consumer behavior demands that businesses adapt their strategies to meet rising expectations for personalized experiences. With the integration of advanced technologies like artificial intelligence (AI) and virtual reality (VR), marketers are poised to create more immersive and tailored interactions than previously imagined. The emphasis on ethical personalization, driven by privacy-first principles, adds another layer of consideration, reflecting consumers’ desire for control over their data while still benefiting from customized experiences.
These technologies allow brands to craft experiences that resonate with individual preferences, transforming how consumers interact with products and services. For instance, VR can offer virtual try-ons or immersive product demonstrations, creating a more engaging way for customers to experience a brand before making a purchase. This combination of AI-driven insights and immersive experiences has the potential to elevate brand loyalty by creating meaningful connections.
At the same time, the rise of personalized video content represents another frontier in digital marketing. Videos tailored to the viewer’s interests, behaviors, and demographics can significantly enhance engagement rates. Research shows that personalized video content can lead to increased viewer retention and conversion rates, as it resonates more deeply with audiences. Companies are increasingly leveraging data analytics to create video campaigns that speak directly to consumer needs and desires, leading to higher satisfaction and engagement.
Conversational commerce is also on the rise, facilitated by personalized chatbots that offer tailored assistance and recommendations. These AI-powered tools can engage customers in real-time, providing personalized interactions based on previous purchases and preferences. This not only enhances the customer experience but also drives sales through seamless, context-aware conversations.
As consumers become accustomed to these interactions, businesses that integrate conversational commerce into their digital strategies are likely to see improved customer satisfaction and loyalty. However, as brands strive for deeper personalization, they must also navigate the ethical implications of their practices. The increasing focus on privacy-first marketing emphasizes the need for transparency and consumer consent. Organizations are recognizing that responsible data usage is essential for building trust with consumers. By adopting ethical personalization strategies, businesses can ensure they respect user privacy while still delivering relevant, tailored experiences.
Therefore, the future of personalization in digital marketing is bright yet complex, driven by technological advancements and an evolving understanding of consumer expectations. Brands that can effectively integrate AI, VR, personalized video content, and conversational commerce while prioritizing ethical practices will be well-positioned to thrive in this new era. As we move forward, the challenge lies in balancing personalization with privacy, ensuring that marketing strategies resonate with consumers while respecting their data rights.
Conclusion
Personalization in digital marketing has emerged as a fundamental strategy for creating meaningful customer experiences and driving business success. As marketing evolves, the shift from traditional mass marketing to individualized approaches highlights the need for brands to understand and anticipate the unique preferences of each customer. By leveraging data analytics, artificial intelligence, and advanced technologies like Customer Data Platforms, companies can deliver tailored content and offers that resonate on a deeper level, leading to higher engagement, increased conversion rates, and improved customer loyalty.
However, the benefits of personalization come with challenges, such as ensuring data privacy, managing technological complexity, and avoiding over personalization. To navigate these obstacles, marketers must adopt ethical data practices, prioritize transparency, and embrace a customer-centric approach that respects individual boundaries. The future of personalization will likely involve greater integration of AI, virtual reality, and hyper-personalized experiences across multiple channels, demanding a balance between innovation and responsible data usage.
In an increasingly digital world, companies that effectively implement personalization strategies while upholding ethical standards will be well-positioned to thrive. As consumer expectations continue to grow, personalization will not only be a competitive advantage but a necessity for delivering relevant and valuable marketing experiences.
Table of Content
- Everything About Personalization in Digital Marketing / Part 1
- Everything About Personalization in Digital Marketing / Part 2
- Everything About Personalization in Digital Marketing / Part 3
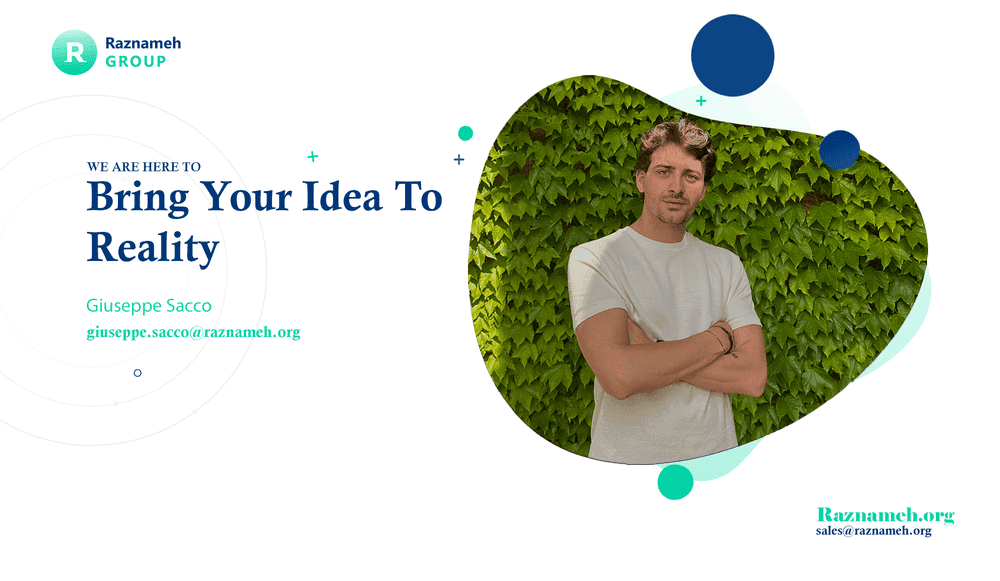